I’ve recently had several questions about how to select and use AI tools for L&D, particularly in the content space. I also regularly see posts about the democratisation of AI (e.g. this, by Erica Farmer) which is a very important driver in the AI revolution. Generative AI content development is not a rabbit hole I usually enter in public, more something I see as an enabler to my business, or for specific strategic discussions with clients. However, with all the debate about the new Studio Ghibli replication by OpenAI, I felt inspired to write about it today.
I’m not going to go into the back and forth of copyright, my position is that training data should be used with creator consent. I don’t know whether the anime stylings of Spirited Away that I referenced over the last few weeks in podcasts and speeches (first in an upcoming podcast with Taryn Hess, Ph.D. and Mike Cooke and secondly at Women In Change’s event last week with Louise Robey) were generated through Studio Ghibli consenting to having their works used as training data. But creator consent is the ideal scenario. Anyway, to the meat of the article.
Corporate learning departments have long faced a challenge: creating visually stimulating and engaging content that resonates with learners whilst managing tight budgets and timelines. Traditional approaches typically involve purchasing generic stock photos or commissioning expensive custom illustrations, both options presenting significant compromises. Stock photos often lack relevance to specific learning scenarios, whilst custom illustrations require substantial time and financial resources. This constraint has led to balancing efficiency with creativity (often efficiency wins), where learning designers settle for “good enough” visuals rather than truly impactful imagery that enhances learning outcomes.
I’ll write this article from the perspective of using Midjourney, Reve, Sora, and Gemini, all AI-powered image generation tools that I can use within my AI ecosystem. There are other image generators available, such as Dall-E (by OpenAI), Adobe Firefly, and Leonardo, which I don’t use directly for various reasons, or only use via API. Choose a tool that works for you: most of the tools on the market fundamentally work in a similar way from the user experience perspective. By translating text prompts into high-definition, customised visuals in minutes, these tools offer corporate learning teams an unprecedented opportunity to prioritise creativity without sacrificing efficiency, as an alternative to stock footage.
The latest updates to apps (particularly Reve and ChatGPT are able to generate accurate text as well, so expect a flood of whiteboard writing images across your socials in the coming few weeks, alongside a flood of anime images of people.
Transforming Corporate Learning Through AI-Generated Visuals
Text-to-Image generation works by interpreting detailed text descriptions and generating corresponding images based on each model’s training data. For instance, a learning designer could request “a diverse team collaborating in a modern office space, solving a complex problem on a digital whiteboard” and receive multiple realistic image options within minutes, each uniquely created based on that specific prompt.
This capability addresses several critical needs in corporate learning:
- Relevance and Customisation: Rather than searching through thousands of stock photos hoping to find something “close enough”, learning teams can generate exactly what they need. This ensures that visual elements precisely match learning objectives and organisational contexts.
- Representation and Inclusion: Learning materials can now easily feature diverse characters, scenarios, and environments that reflect an organisation’s global audience, without the limitations of existing stock photography collections.
- Cost-Effectiveness: Whilst premium AI image generation services require subscription fees, they’re typically far less expensive than commissioning custom illustrations or purchasing extensive stock photo collections, especially when calculated on a per-image basis.
- Rapid Iteration: Learning designers can quickly experiment with different visual approaches, or variations on a theme, generating multiple versions of concepts to test with stakeholders before finalising content. This is particularly helpful if designers want to work with agentic workflows or automation for rapid prototyping.
Example: Images for a Corporate Presentation Scene
This short article is not intended to give a detailed view on bias or even to produce finished media without any AI artefacts, but to illustrate prompt structure and the nuance of specific technical parameters, and to give some measure of side-by-side comparison between tools. I’ve deliberately kept the prompts short and simple to use the same text across multiple generators.
Here I’ll use Reve (a free generator that gives 1-8 images per prompt); Midjourney 6.1 Standard (paid license, 4 images per prompt); Sora (OpenAI pro account (1-4 images per prompt); Gemini 2.0 Flash (free usage, 1 image per prompt).
Notably, although OpenAI has announced that ChatGPT has 4o image generation, all prompts below returned a failure to produce an image via that route.
Prompt 1 – Basic with Style:
“professional man in navy blue suit presenting in modern corporate boardroom, data visualisation projected on screen behind him, cinematic lighting, ultra realistic –ar 16:9 –stylize 25”
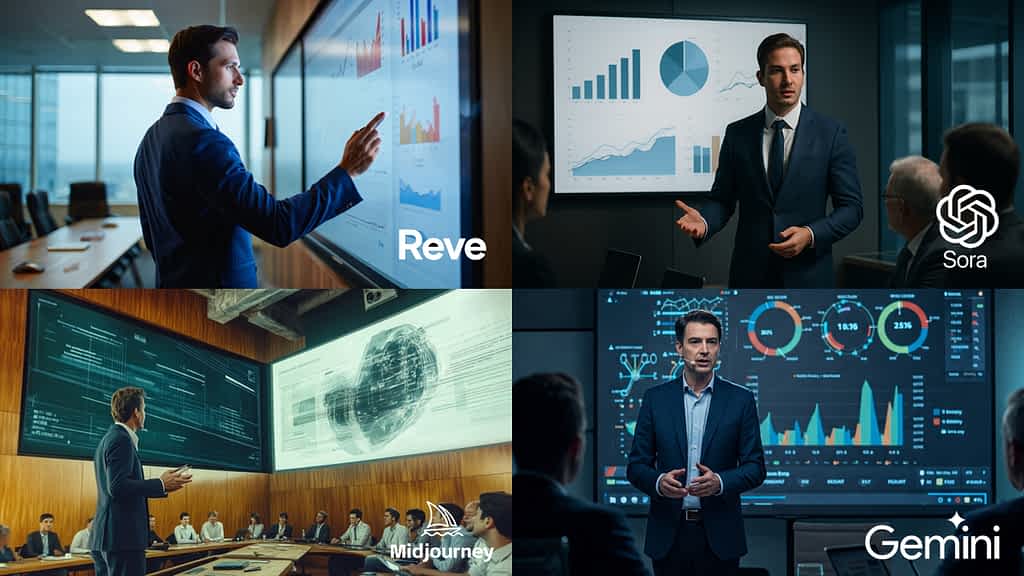
Midjourney technical parameters explained: –ar 16:9: Sets the aspect ratio to widescreen format, ideal for presentation slides –stylize 25: Controls the artistic interpretation (range 0-1000, where lower values maintain photorealism and higher values create more artistic effects)
Gemini and Sora don’t return 16:9 images, so these are cropped here.
Prompt 2 – Detailed with Atmosphere:
“executive presenting financial data, wearing tailored cerulean suit, glass-walled boardroom, holographic charts floating behind, morning sunlight streaming through windows, high-end corporate environment, photorealistic, 8k –chaos 20 –ar 16:9”
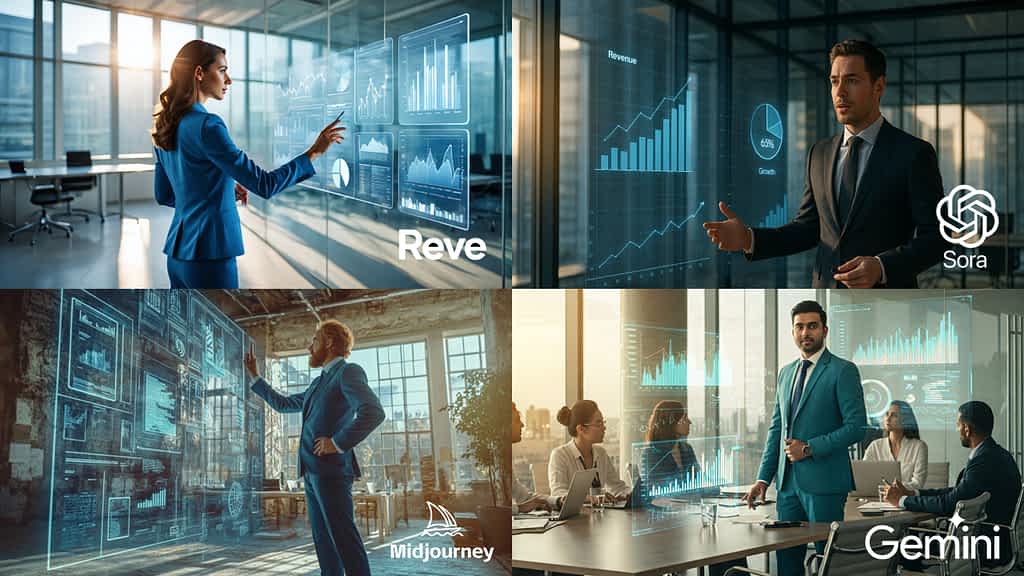
Technical parameters explained:
–chaos 20: Controls image variability/unexpectedness (range 0-100, where higher values produce more surprising and unique results)
–ar 16:9: Sets the aspect ratio to widescreen format
Midjourney technical parameters explained: –chaos 20: Controls image variability/unexpectedness (range 0-100, where higher values produce more surprising and unique results) –ar 16:9: Sets the aspect ratio to widescreen format
Notably, Sora and Reve return text that is readable in English without spelling error.
Prompt 3 – Creative with Technical Parameters:
“business presenter gesturing towards analytics dashboard, sapphire blue Tom Ford suit, minimalist conference room, dramatic rim lighting, volumetric fog, inspired by Greg Williams photography –stylize 75 –v 5.2 –niji 5”
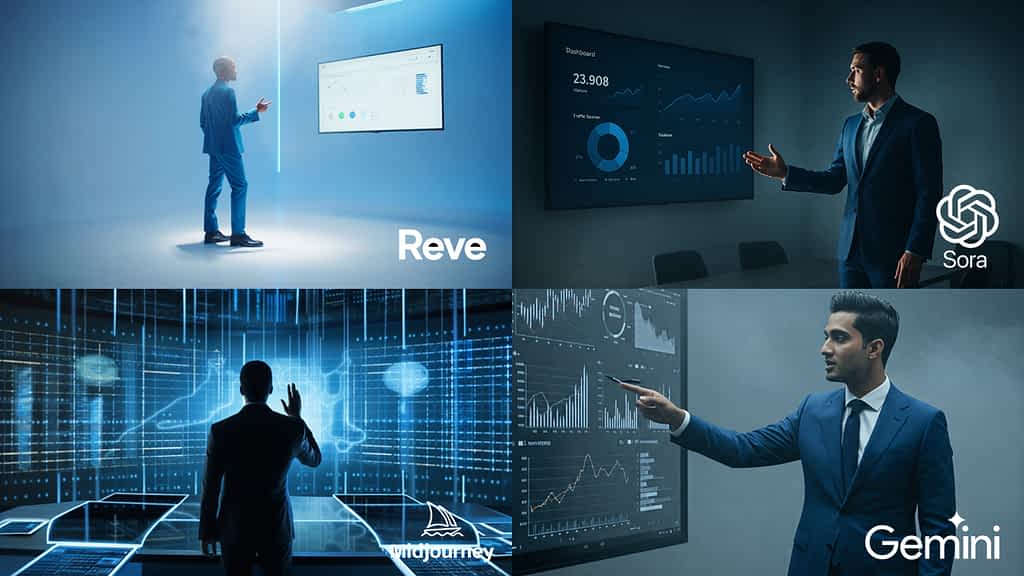
Midjourney technical parameters explained: –stylize 75: Higher stylisation value creates more artistic interpretation –v 5.2: Specifies the Midjourney model version being used –niji 5: Activates Midjourney’s anime/illustration-focused model for a more stylised look
Sora failed on this prompt until references to Tom Ford and Greg Williams were removed. All other generators yielded an image.
From Technical Execution to Creative Strategy
Image generation technologies shift the focus of learning designers from technical execution to creative strategy. Rather than spending hours searching through stock libraries or managing illustration vendors, designers can devote their expertise to conceptualising the most effective visual approach for learning objectives.
The key skill has been termed “prompt engineering”: the ability to craft detailed, specific text descriptions that yield the desired images. Learning professionals who master this skill can produce visuals that were previously unattainable without specialised design expertise or significant budgets.
Prompt engineering was a popular buzzword over the last couple of years, but scripting prompts manually might be less important in future as more platforms have built-in presets which contain detailed prompt structures, relieving users of the burden of creating prompts, or as large language models (LLMs) are able to create detailed prompts for designers to use in their image generator of choice.
The transformative potential of AI image generation extends far beyond static visuals. These generated images can serve as foundational assets for creating rich, interactive learning experiences. When an AI-generated image is created, it can become the starting point for developing dynamic video content through image-to-video tools (e.g. Invideo, Flux, Runway, Haliuo, Kling), constructing immersive 3D environments for virtual training scenarios, or creating augmented reality experiences. This capability democratises multimedia content creation, allowing L&D teams to produce sophisticated learning materials that were previously cost-prohibitive or technically out of reach. For instance, a single generated image of a manufacturing process could be transformed into an animated sequence, integrated into a virtual reality training simulation, or used as the basis for an interactive 3D model, all without the traditional overhead of professional video production or 3D modelling expertise (one method is ZoeDepth).
Note: with the market moving rapidly, many video creation tools are able to generate photorealistic video directly from text prompts, so the choice to use seed images is primarily stylistic.
Implementing AI Image Generation Responsibly
Organisations embracing this technology should consider several implementation factors:
- Skills: Learning designers should have structured opportunities to experiment with AI image generation and develop the necessary skills specific to using the tools that are available within their technical ecosystem.
- Ethical Guidelines: Establish clear policies regarding appropriate usage, considering factors like image authenticity, avoiding stereotypes, and maintaining organisational values.
- Integration with Workflows: Determine how AI-generated visuals will complement existing design processes rather than simply adding another tool to an already complex workflow.
- Copyright and Ownership: Understand the terms of service for AI image generation platforms regarding image ownership and usage rights within learning materials.
Workflow Integration: Automating Image Generation
Whilst manual image generation through Midjourney’s interface is powerful, true scalability comes through workflow automation. Low-code and no-code platforms offer exciting possibilities for integrating AI image generation directly into existing learning development processes.
Modern automation platforms have revolutionised the way teams can implement AI image generation at scale. Through platforms like Make.com and n8n, learning teams can create sophisticated automated workflows that trigger image generation based on content updates, seamlessly integrate with learning management systems, and even facilitate automated image variation testing for e-learning modules.
These automation platforms serve as powerful connectors, enabling learning teams to build workflows that link APIs with their existing technology stack. Midjourney has no easily accessible API for automation at the time of writing, but others like Leonardo.ai are available. Your tool choices should reflect your needs, so research tools before you buy something. Content management systems, project management tools, asset libraries, and collaborative design platforms can all be connected through these automation tools, creating a cohesive ecosystem for visual content creation.
The real power of these integrations lies in their ability to democratise AI image generation across entire learning and development teams. By reducing manual effort and establishing consistent processes, teams can focus more on creative strategy and less on technical implementation. This systematic approach ensures that AI-generated visuals become a seamless part of the content creation pipeline rather than a separate, siloed process.
Looking Ahead
As AI image generation technology continues to evolve rapidly, its potential applications in corporate learning will expand, both in creating static visuals, and as a seed for immersive learning experiences. Organisations that gain experience with these tools will be best positioned to leverage increasingly sophisticated capabilities as they emerge.
The real promise lies not just in more efficient visual content creation, but in fundamentally reimagining what’s possible in corporate learning experiences. When visual constraints are removed, learning designers can focus on creating truly transformative educational experiences that drive organisational performance.
Ready to explore how AI can transform your learning organisation? Visit www.mehtadology.com to learn more about being part of the AI revolution and discover practical approaches to implementing these technologies within your learning ecosystem.