Corporate learning has long relied on SCORM (Sharable Content Object Reference Model) data to track employee progress. Whilst this approach has served us well for years, it primarily captures surface-level metrics like completion rates and test scores. As organisations embrace more complex learning environments, these limited data points are no longer sufficient to truly understand learning effectiveness.
SCORM (Sharable Content Object Reference Model) is a set of technical standards for e-learning software products. Developed in 2000, it defines how online learning content and Learning Management Systems (LMS) should communicate. SCORM enables learning content to be packaged into a transferable ZIP file that can be uploaded to any SCORM-compliant LMS, ensuring compatibility and standardized tracking of basic learning metrics like completion status, time spent, and assessment scores.
The Limitations of Traditional Learning Analytics
Traditional learning management systems excel at answering questions based on rudimentary time-stamped completion data, which is good enough for a completion tick, but doesn’t help us to reshape learning or teaching strategies beyond that. SCORM data helps us to answer questions: Did the employee complete the course? What score did they achieve? How long did they spend on each module? Whilst valuable, these metrics fail to capture deeper insights about the learning experience itself, how learners were engaged, and what questions arose through the learning experience.
SCORM data tells us what happened but rarely explains why it happened or how the learner felt during the process. This creates a significant blind spot for learning and development teams trying to optimise training programmes and drive meaningful outcomes.
The AI-Powered Evolution
Generative AI (particularly large language models used through conversational agents) is fundamentally changing this landscape. Through sophisticated sentiment and semantic analysis, these systems can generate data that allows learning professionals to identify patterns in learner queries, detect emotional responses to content, and map common areas of confusion or misunderstanding.
As organisations increasingly incorporate AI-powered virtual agents into their learning ecosystems, they’re able to gain access to a wealth of conversational data that far exceeds traditional SCORM metrics in both depth and utility. Natural language processing can reveal not just what learners are asking about, but also their confidence levels, engagement, and even potential misconceptions about the material.
Understanding AI Agents vs Assistants in Learning
AI Agents operate autonomously in learning environments, working proactively in the background to monitor progress, make decisions, and initiate actions without constant user input. They can automatically adjust content difficulty, identify learning gaps, and implement interventions across thousands of learners simultaneously.
AI Assistants function as responsive support systems, operating on a request-response model. They wait for learner queries before providing help, explanations, or guidance. Their strength lies in delivering immediate, contextual support while maintaining a complementary role to human instruction, making them ideal for direct learner engagement.
Using conversational AI technologies (from chatbots and virtual agents to pedagogic assistants) can go beyond just delivering content, to support learners by engaging in dialogue, asking and answering questions, providing explanations, and supporting learners throughout their journey. Every interaction creates valuable data that can be analysed for insights that were previously impossible to capture.
From Completion Rates to Conversational Intelligence
This shift from basic completion metrics to conversational analytics enables several transformative capabilities:
- Real-time support: AI can identify confusion or frustration in a learner’s language, allowing for immediate intervention rather than waiting for poor test results.
- Sentiment analysis: Understanding the emotional journey of learners helps identify which content resonates and which creates negative experiences.
- Topic and theme extraction: By analysing learner conversations, organisations can identify common questions, interests, and knowledge gaps across their workforce.
- Learning personalisation: Conversational data reveals individual preferences and needs, enabling truly adaptive learning experiences.
- Content optimisation: Detailed insights about how learners interact with and comprehend material drives continuous improvement of learning materials and resources, similar to A/B testing in marketing.
A/B testing in social media marketing is a systematic method of comparing two versions (A and B) of content, advertisements, or campaigns to determine which performs better. Marketers create two similar variations of content, showing version A to one segment of their audience and version B to another. By analysing metrics like engagement rates, click-throughs, conversions, and audience response, teams can make data-driven decisions about which content strategies are most effective. This approach helps optimise everything from ad copy and imagery to posting times and content formats, leading to improved ROI on social media marketing efforts. The insights gained from A/B testing enable continuous refinement of marketing strategies based on actual user behaviour rather than assumptions.
Preparing for the Transition
Organisations looking to leverage these advanced analytics capabilities should consider several key factors:
- Skills Development: L&D teams will need to develop new competencies in data analysis and AI literacy. The ability to interpret conversational insights and translate them into actionable improvements becomes crucial.
- Ethical Governance: The collection and analysis of conversational data raises important privacy considerations. Organisations must establish clear policies about data usage, transparency, and employee consent.
- Technology Infrastructure: Moving beyond SCORM requires investment in platforms that can capture, analyse, and visualise conversational data. This may involve integrating new tools with existing learning ecosystems, or discussing data and conversational capabilities with existing providers.
- Cultural Readiness: Both L&D professionals and learners need preparation for more interactive, conversation-based learning experiences. This represents a significant shift from traditional e-learning approaches, and may need a reconfiguration of success criteria, KPIs, business cases, to re-evaluate how learning departments are measured, incentivised, and demonstrate return on investment.
Mehtadology’s AI Readiness Framework
The four pillars discussed above – Culture, Skills, Governance, and Technology – form part of Mehtadology’s AI Readiness Framework. This comprehensive approach helps organisations systematically assess and build their capabilities for successful AI implementation. By addressing these interconnected elements strategically, organisations can create a solid foundation for AI adoption while minimizing risks and maximizing value creation.
Figure 1: Mehtadology’s AI Readiness Model
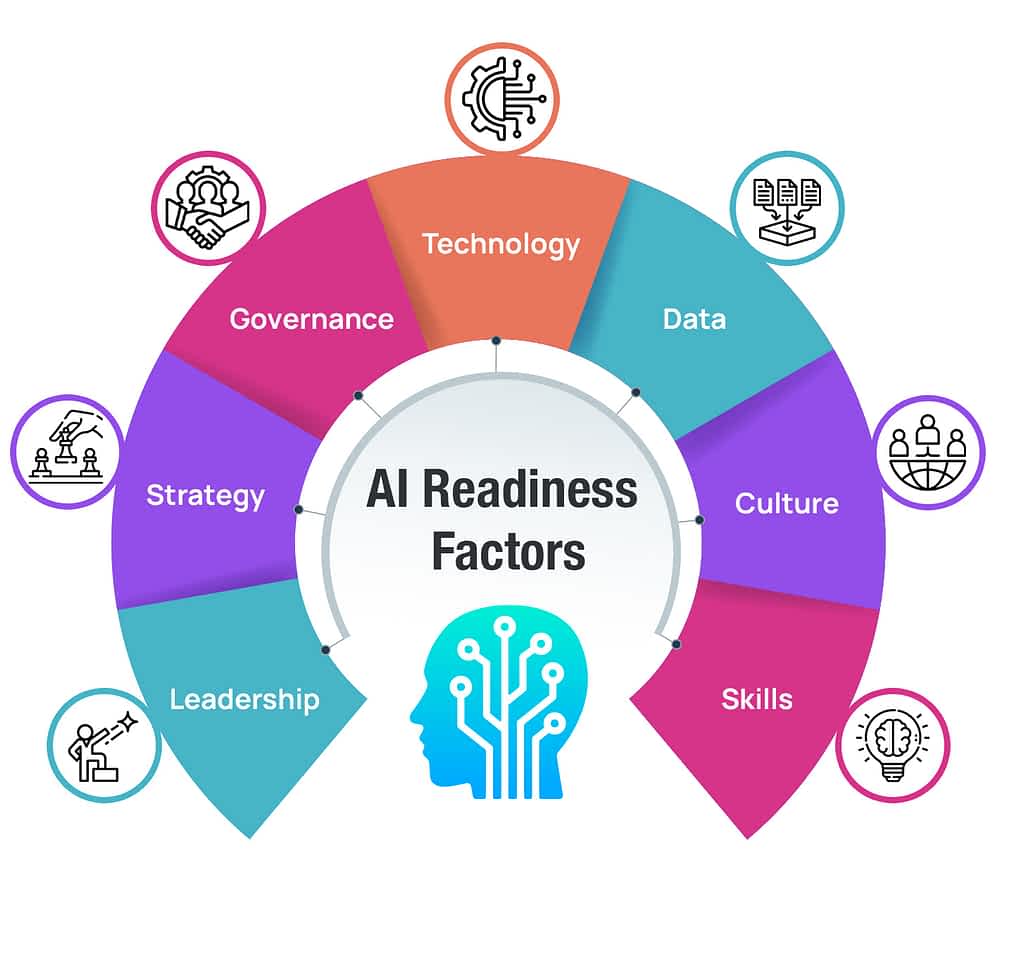
The Future of Learning Analytics
As generative AI becomes more sophisticated, we can expect even greater advances in learning analytics. Future systems will likely combine conversational insights with other data sources, including performance metrics, career progression, and maybe even demographic or personal information to create holistic views of learning effectiveness.
Organisations that embrace this evolution will gain significant advantages in developing their workforce through targeted interventions, moving to personalisation of learning (rather than role-based customisation of content), closing skills gaps, and creating more engaging, effective learning experiences.
The transition from SCORM to conversational analytics isn’t just a technical upgrade, it’s a fundamental reimagining of how we understand, measure, and improve corporate learning and performance.
To learn more about incorporating AI-powered analytics into your learning strategy and being part of the AI revolution in human capital management, explore www.mehtadology.com.